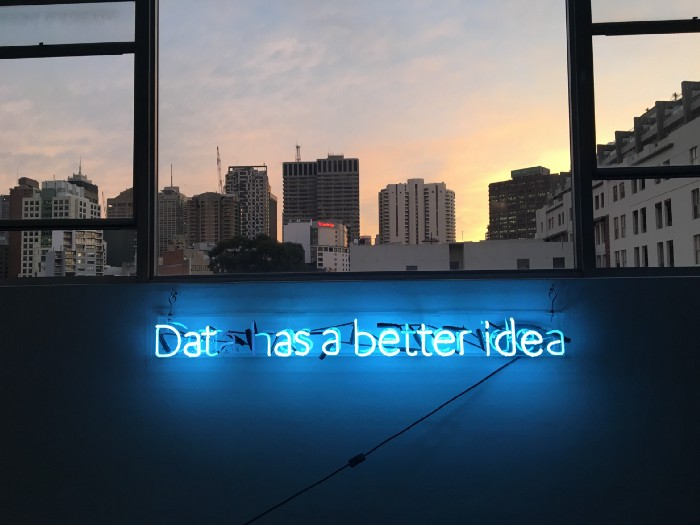
What happens when computers know people better than they know themselves?
Science is an algorithm. To date, it might well be the most effective and useful algorithm — or family of algorithms — that humanity ever invented. Ever-improving methods for erecting models of how the world works and then testing those models against evidence make it possible to distinguish good ideas from bad. Step-by-step, humanity’s understanding of the universe, the world, and itself, has grown. The Artificial Intelligence revolution, however, could well overturn how good ideas are sifted from bad and subvert science’s ultimate goal of understanding.
The claim that AI could undermine scientific understanding, or even make it obsolete, is far from new. Deep learning algorithms, unlike scientific ones, care not a bit about understanding. They optimize more proximate and banal quantities. More than a decade ago, Chris Anderson argued that ‘the data deluge makes the scientific method obsolete.’ He predicted the end of scientific theory.
The new availability of huge amounts of data, along with the statistical tools to crunch these numbers, offers a whole new way of understanding the world. Correlation supersedes causation, and science can advance even without coherent models, unified theories, or really any mechanistic explanation at all.
In 2017, David Weinberger stirred things up againwhen he asserted that ‘our machines now have knowledge we’ll never understand.’ Whether accounts of theory’s death have been exaggerated or not remains a topic of vigorous, sometimes heartening debate. Peter Sweeney suggests that the new and inexplicable insights that arise from deep learning might provide a renaissance for deeper explanations, concluding that:
Deep learning should be celebrated, not for revealing the limits of knowledge, but as a powerful observational tool.
The proper study of humankind
Most discussions about deep learning supplanting the scientific method orbit around the natural sciences and medicine. From astronomy and particle physics to genomics, bioinformatics, and disease modeling, it doesn’t take enormous feats of imagination to see how deep learning algorithms might mine the vast seams of available data. It matters, however, whether this mining effort exposes new gems of understanding, or simply a rich ore of profitable predictions. When the claims are staked in the soil of the social sciences, however, it matters a great deal more.
Distilling knowledge about human behavior and society from the murky slurry of folklore, wishful thinking, and belief grows altogether more difficult than understanding the physical universe. For millennia, progress toward evidence-based understandings of human behavior and society have been fouled by self-interest, moral intuition, religious teachings, political dogma, and our idiosyncratic upbringings. Progress suffers, too, from a not-uncommon view that science has no place in humanity’s understanding of itself. Even academic disciplines that claim to use scientific methods tend to agglomerate into tribes, each driven to prove the rightness of their ideas.
Deep learning is poised to put an end to those squabbles. Not that it will clarify the murk and finally reflect back to us who we are and how we become ourselves. Instead, deep learning algorithms will extract, from the mountains of user data, clicks, likes, follows, tweets, messages, purchases, and movements, everything they need in order to sell products, win votes, and shape opinions. Artificial intelligence could displace human understanding and all the many competing, incompatible, and mutually contemptuous forms of ‘theory.’ Yes, even Critical Theory.
Nature and nurture
Ultimately, all conflicting theories about humanity begin at the watershed between nature and nurture. Eventually, they each return, like migrating salmon, to one of these natal streams, again to perpetuate the false dichotomy that has obscured human understanding for as long as people have thought seriously about how they came to be who they are. Plato and Aristotle argued about whether ideas arise innately or through experience, and the same question has occupied philosophers more than any other.
In the twentieth century, things grew especially hostile. Pioneering cultural anthropologist Alfred L. Kroeber asserted, in 1915, that ‘Heredity cannot be allowed to have acted any part in history.’ Biological/psychological explanations could never be reconciled with social/historical studies. Generations of anthropologists took Kroeber at his word, and, to be fair, they had reason. The eugenicists and social Darwinists had already shown the catastrophe of inferring what ought to be from their blinkered and wishful views of how nature works. The Nazis were soon to demonstrate just how brutally ideas about innate human differences and racial superiority could be coopted to abusive ends.
Today, the term ‘evolutionary psychology’ can induce spittle and rage in otherwise measured researchers, convinced that any biological explanation for human behavior inevitably forges the thin end of the next Fascist wedge. In return, this reception induced paranoia in many evolutionary psychologists, many of whom are convinced that a hegemonic ‘Standard Social Science Model’ imposes naive but politically palatable ideas that humans begin life as blank slates, shaped only by socially constructed or cultural factors.
Similar battles rage within anthropology, wherein the so-called ‘theories’ of biological and cultural anthropologists are often mutually unintelligible to the other tribe. Understanding nature, nurture, and the complicated ways in which they interact is important for anybody who wants to understand where inequity, violence, and exploitation come from and then do something about it. Sociology, psychology, and economics all falter under the weight of the commitment to theories that champion one cause and denigrate or ignore the other.
Unless all the social sciences move beyond their Babel-like tribalism and self-insulation, they will be rendered irrelevant by AI. The people and corporations interested in how humans interact, consume, and spend have little interest in the extent to which the behaviors arise via inheritance or experience. Algorithms have no use for explanations, much less the ornate but trivial part-explanations that so many researchers hopefully call “theory”.
The end
The example of children learning to read throws some of the issues into relief. Reading requires the repurposing of neurological pathways that evolved to fulfill other functions. Many difficulties in learning to read have a biological component to them. Despite the fact that it takes both nature (nervous systems) and nurture (learning) to become a reader, the old childish fight over which is most important continues to rage.
The most unseemly fight — known simply as the ‘reading wars’ — runs at a tangent to the nature-nurture fault line. It concerns two different models of nurture: systematic phonics, which teaches associations between letters and sounds, and whole language, in which students infer or guess, from pictures, context, and other cues, the words that they don’t recognize in written form. Although some researchers suggest blending the methods or finding better approaches, many prefer to persist in vicious squabbles that would surely have any first-grader sent to the naughty corner. Or whatever ‘best-practice’ says to do these days.
Studying how best to teach children to read is genuinely hard. To begin, you have to design a study that offers some children phonics teaching, and other children whole language, in a way that is fair to both methods. You then need to recruit a lot of children and get their guardians’ and teachers’ consent to their participation. Then the hard work begins, watching the children learn, and measuring relevant outcomes, preferably over several years. Even if you could do all those things, would it be ethical to persist if it started to look like one method was more effective than the other? Could you prevent parents and relatives from using the other method at home?
It would take millions of dollars to do all of the steps properly and ethically. That’s only for one language in one cultural and economic context. Add to this the wasted hours applying for and missing out on grant funding before the scientist ever sees their first dollar of research support.
Artificial Intelligence offers a tantalizing alternative. One could, at least in theory, build software that teaches children to read via any chosen method, measures their progress, and evaluates the success of each approach. But why stop there? With only a little more work, one could build the software to learn from children even as it helps them learn to read. It could generate new strategies that combine the best that phonics, whole language, and any other method of teaching have to offer.
Such yet-to-be-built software might sort out the doctrinaire ‘phonics’ versus ‘whole language’ squabble, coming down decisively on one side. More likely, however, it would converge on new strategies for teaching children to read that don’t chime with either of the warring theories. Indeed, the strategy might well defy description entirely. Children might learn how to read in ways that they enjoy more, that are faster, and that frustrate them less than any previous generation of learners, but nobody will be able to understand how.
This is the kind of outcome we might expect if David Weinberger was right when he wrote that:
We are increasingly relying on machines that derive conclusions from models that they themselves have created, models that are often beyond human comprehension, models that “think” about the world differently than we do….
… Knowing the world may require giving up on understanding it.
Would educators or parents accept this kind of future? Even if the children become better, more enthusiastic readers than any previous generation? For one thing, if deep learning learns how best to teach children to read, it won’t be the product of some benign public-good scheme to improve the store of human knowledge. Not with the way research and education funding have been traveling in recent decades. Instead, private companies will develop the software, accumulate the data, and benefit from whatever their algorithms learn.
Wealthy parents will be able to afford the best that the new technologies can offer their reading-age children. Other parents won’t. If you thought the ideological battles over how to teach reading were vicious, you could get a foretaste of the future by studying the firestorms that engulf private-versus-public schooling.
Divorce your tribe
Data that could render obsolete all of the difficult, ideologically-charged issues surrounding human behavior and society are out there already. More accumulate by the day. Yet they won’t necessarily improve our understanding of one another and how we function. I believe the stakes are far too high for the social sciences and human self-knowledge to fall into the unknowable abyss of deep learning.
If social science is to stay relevant, it has to develop a renewed and laser-sharp focus on the ‘science’ part. Anybody interested in understanding what humans are really like, beneath all those layers of culture, socialization, genetics, epigenetics, privilege, wealth, and power, will need to divorce their own tribe and seek citizenship of the entire scientific world. Only then can they hope to build on those few ideas that hold up under evidence and to reject those that don’t.
We need to improve the social-scientific algorithm. To do so, we will need to finally shed our technicolor dream coats of wishful thinking and find disinterested ways of sifting evidence and learning from the masses of available big data. A new social science that has as much access to data as corporations do, and that embraces deep learning, stands a chance of improving both our understanding of ourselves and all human lives.
If social scientists can’t do these difficult things, then machines, and the corporations that own them, will learn whatever they like about us, knowing us but still not understanding a thing. And they will make a killing.
…
This article was first published in Towards Data Science on the 1st October 2020.
Join my mailing list for monthly updates.